Lupine Publishers |Agriculture Open Access Journal
Abstract
The study analyzed the household poverty status of small scale farmers in Bayelsa State, Nigeria using a multi-stage random sampling technique to sample six hundred farmers. Data were collected using structured questionnaire and were analyzed using descriptive statistics, FGT [1] index and the logistic regression model. The result revealed that the majority of the farmers 80% were females, while 79% of the respondent was married with 46% of them having no formal education. Twenty-seven (27) percent of the crop farmers are poor while thirtyeight (38) of the livestock farmers were poor. Also, the poverty depth and severity of crop farmers were 0.072 and 0.038 respectively whereas they were 0.098 and 0.052 respectively for the livestock farmers. The logistic regression model revealed that age, educational level, household size, farming experience, farm/herd size, household income, household expenditure and membership of cooperative contributed significantly in determining the poverty status of the farmers. This study therefore recommends measures needed to be put in place to encourage and improve the welfare of the farming household towards productive and sustainable agricultural development for poverty reduction.
Keywords: Economic; Poverty; Status; Small Scale; Farmers
Introduction
Nigeria is a vast country endowed with substantial natural resources which include; 68 million hectares of arable land, fresh water resources covering about 12 million hectares, 960 million hectares of coastline and ecological diversity that favor the production of a wide variety of crops, livestock, forestry and fisheries product [2]. These coupled with its 37 million hectares of natural forest and rangeland and total land mass of 923,768km2 [3] makes agriculture one of the prominent sub-sector. In spite of these resources’ endowment, the productivity of agriculture continues to dwindle. One of the major problems confronting Nigeria today is how to improve the quality of life in the rural areas and reduce the level of poverty [4]. Poverty in Nigeria is not only a state of existence but also a process with many dimensions and complexities [5]. The report of the 2006 Nigerian Core Welfare Indicator (CWI) on the poverty profile in the country stated that the dependency ratio, which was defined as the total number of household members aged 0 – 14 years and 65 years and above to the number of household members aged 15 – 64 years was 0.8 Central Bank of Nigeria [6]. This indicated that almost a one-to-one dependency ratio and reflected the high population growth rate in the country. There is also large income inequality with the top 10% of the income bracket accounting for close to 60% of the total consumption of goods and services [7].
The World Bank [8] describes poverty to comprise of many dimensions. It includes low incomes and the inability to acquire the basic goods and services necessary for survival with dignity. It encompasses low levels of health and education, poor access to clean water and sanitation, inadequate physical security, lack of voice and insufficient capacity and opportunity to better one’s life. It may also result in not having enough capacity to feed and clothe the family and/or earn a living. About 90% of the country’s food is produced by small scale farmers cultivating tiny plots of land who depend on rain fed agriculture [9]. According to Omonona [10], poverty is pervasive although the country is rich in human and material resources that should translate into better living standard. Despite its plentiful resources and oil wealth, poverty is widespread in Nigeria [11]. The situation is said to have worsened since the late 1960s, to the extent that the country is now considered one of the 20 poorest countries in the world. Over 70% of the population is classified as poor, with 35% living in absolute poverty. Poverty is especially severe in the rural areas, where social services and infrastructure are limited or non-existent. Majority of those who live in rural areas are poor and depend on the agriculture for food and income.
The concern on the threat posed by poverty has led the Nigerian government over the years to devote considerable attention to alleviating its scourge through various policy projects and programmes which seems not to have stem the ugly situation till date. In view of these, the question about the poverty status of rural dwellers especially the small scale farmers remained unanswered. It is on this premise that this study was carried out to answer these questions.
a) What are the socio-economic characteristics of these small scale farmers?
b) Are the small-scale farmers really poor?
c) What are the factors influencing poverty status of the small scale farmers? and
d) What options are available to small scale farmers in reducing their poverty levels?
Thus, the main objective of this study is to evaluate the poverty status of small scale farmers in Bayelsa State, Nigeria. The specific objectives are to:
a) Describe the socio-economic characteristics of small scale farmers.
b) Compare the poverty status of crop and livestock farmers in the study area;
c) Determine the factors influencing poverty status of the farmers; and
d) Make policy suggestion towards poverty alleviation.
Methodology
Study Area
The study was conducted in Bayelsa State, Nigeria. It is located between latitude 5° 001 to 10° 301 N and longitude 4° 551 to 6° 001 E and covers an estimated land area of 1,810km2 with a population of about 856,729 thousand [12]. It shares local boundary with Delta State, Anambra State and Rivers State with the Bight of Benin at the Southern Flank. There are eight (8) Local Government Areas (LGAs) in the state with Ijaw as their major language. Mean annual rainfall of the area is 2,200mm for upland or dry regions where water bodies are few and 3,500mm for wetland or lowland region which comprises of land areas being surrounded by water bodies. Temperature range is between 23 – 31°C and vegetations found in the area include the saline water swamp, mangrove swamp and the rain forest. Major seasons are the dry (November – February) and wet seasons (October – March). Also, the seasonal condition of the area presents a healthy environment for farming which is the main source of income and livelihood of the state’s population and agriculture accounts for about 72% of the labor force.
Sampling Procedure and Sample Size
The sampling involved a multistage random sampling technique. Firstly, six (6) local government areas were randomly selected using the proportionate sampling method at 75% precision level from the purposively selected three (3) agricultural zones according to Agricultural Development Programme (ADP) structure. In the second stage, ten (10) villages were also randomly selected from the six (6) LGAs each making a total of sixty (60) villages. The third stage involved a simple random selection of five (5) crop and five (5) livestock farmers each from the villages using the list provided by ADP from each of the villages. A total of six hundred (600) respondent farmers were used.
Data Collection
Both primary and secondary data were used for the study. The collection of primary data was achieved using a set of structure questionnaire that was administered by the researcher and trained enumerators complemented with oral interview, information that was collected covered the areas of socio–economic characteristics, farming operations, and income and expenditure patterns. Secondary data were sourced from relevant material both published and unpublished.
Analytical Technique
Three analytical tools namely; descriptive statistics; Foster, Greer and Thorbecke (FGT) model of poverty decomposition [1]; Logistic regression were used for the study. Descriptive statistics such as frequency, percentages, mean were used to profile the socio-economic characteristics of the farming households and also to present the results of the findings. The FGT measure was used to assess the incidence, depth and severity of poverty of the farming households. The approach makes use of the aggregate values of the poverty indices – poverty headcount, poverty gap, and squared poverty gap. The use of the FGT measures required the definition of poverty line and this was calculated on the basis of aggregated data on household income. The FGT measure as used by Baiyegunhi and Fraser [13] is expressed as:
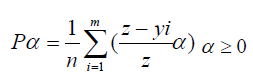
Where:
z = Poverty line
m = Number of households below poverty line
n = number of households in the reference population
yi = Per adult equivalent income of ith household
α = Poverty aversion parameter
z-yi = Poverty gap of the ith household
z – yi = Poverty gap ratio
The headcount index was obtained by setting a = 0, the yield poverty gap index when a = 1, and squared poverty gap index when a = 2. The poverty line is a predetermined and well defined standard of income and value of consumption. In this study, the poverty line was based on the income of the households. A relative poverty line was used in which a household was defined as poor relative to others since they are all farmers. Two third of the mean per capita income (MPCI) was used as a moderate poverty line while one third was taken as the line for extreme poverty. Thus, the farming households were grouped into three categories based on their levels of poverty: the extremely poor (those whose income was less than one-third of MPCI), the moderately poor (those whose income lies between onethird and two-third of the poverty line) and the non-poor (those whose income was above two-third of the poverty line).
Adult equivalents were generated following Nathan and Lawrence [14], thus:
AE =1+ 0.7(N1 −1) + 0.5N2
Where
AE = Adult equipment
N1 = Number of adults aged 15 years and above
N2 = Number of children aged less than 15 years.
Logit Regression Model
A binary logistic regression model was used to analyze the determinants of poverty. Thus, poverty is the dependent variable and is determined by independent variables such as socioeconomic characteristics of households and access to services. The dependent variable is binary (1 if the household is poor and 0 if the household is non-poor). The logit model is based on the cumulative logistic distribution function expressed as:
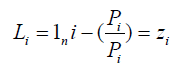
Where:
Li = log of the odd ratio, which is not only linear in Xi but also linear in the parameters,
Pi = is the probability of being poor and ranges from 0 to 1.
Zi = the function of the explanatory variables (x) which is expressed explicitly as:

Where:
Bo = Intercept, Bi – B9 = coefficient of the independent variables, xi = is the vector of relevant independent variables and U = is the stochastic error term, z = the dependent variable defined as the mean annual per capita expenditure. It was measured in binary terms such that 0 = poor, that is if the mean per capita household expenditure is below the poverty line and l = not poor, that is if the mean per capita household expenditure is above the poverty line and:
X1 = Age (number)
X2 = Farm size (number of herds/hectares)
X3 = Marital Status (1 = Married, 0 = otherwise)
X4 = Household size (number)
X5 = Education Level (number of years)
X6 = Major Occupation (1 = farming, 0 = otherwise)
X7 = Farming Experience (years)
X8 = Household income (Naira)
X9 = Household Expenditure (Naira)
X10 = Extension contact (1 = yes, 0 = otherwise)
X11 = Cooperative Membership (1 = yes, 0 = otherwise)
Results and Discussion
Socio-Economic Characteristics of Respondents
Table 1 shows the socio-economic characteristics of the respondents. The majority, 80.3% of the respondents were female while 19.7% were male. This suggest that majority of small scale farmers in the study area are female. About 61% of the respondent were age <30 to 50years with the mean age of 41 years. These results suggest that majority of the farmers were in their active productive age. Moreover, 79.17% of the respondent farmers were married, only 14.83% were single and 6.00% of the farmers were either divorced or widowed. About 46.50% of the farmer does not have formal education, while 32.67% had primary education. Only 16.17% and 4.67% of the respondents had secondary and tertiary education respectively. These result confirm the low level of education in the study area as the state was rated as an educationally disadvantage state in Nigeria. Majority of the respondent farmers 65.67% had a household size ranging between 6 – 10 persons, while 11.50% had less than 5 persons and 22.83% of the respondent had above 10 persons. The result suggests a large household size among the respondent farmers (Table 1).
On the basis of farming experience, about 27.50% of the respondents had less than 10 years farming experience, while 39.50% had farming experience ranging between 11 – 20 years. Only 31.17% had farming experience ranging between 21 – 30 years and 1.83% had farming experience of more than 30 years. Based on household income, about 71.17% of the respondents had annual income ranging between N100,000 – N500,000, while 25.33% had annual income ranging between N501,000 - N1,000,000, only 3.50% of the respondents had annual income above N1,000,000. Majority of the farmers 63.00% had household expenditure ranging between N501,000 – 1,000,000, while 22.83% had expenditure ranging between N100,000 – N500,000 and 14.17% of the farmers had household expenditure above N100,000. These result suggest that majority of the respondent farmers spend more than they earn thereby pushing them more into poverty. Majority of the farmers 85.83% have no access to extension services while only 14.17% of the farmers have access to extension services. Moreso, 65.67% of the respondent farmers are members of cooperative societies while 34.33% do not belong to cooperative society (Table 2).
Analysis of Poverty Status of the Farmers
Table 2 shows the summary of the poverty incidence (P0), depth (P1) and severity (P2) among the respondents. The MPCI of the crop farmers was 21,017.20. This gives a moderate poverty line (2/3 MPCI) of 14,011.47 and a core poverty line (1/2 MPCI) of 7005.73. The MPCI of the livestock farmers was 17,213.10. This gives a moderate poverty line (2/3 MPCI) of 11,475.40 and a core poverty line (1/2 MPCI) of 5737.70. Hence, crop farmers whose monthly per capita income falls between 14,011.47 and 7005.73 were regarded as moderately poor while those who fall below 7005.73 were regarded as core poor and those above 14,011.47 were regarded as non-poor. For the livestock farmers, households whose monthly per capita income fall between 11,475.40 and 5737.70 were regarded as moderately poor while those below 5737.70 were regarded as core poor and those who are above 11,475.40 were regarded as non-poor. The poverty incidence (Table 2) shows that among the crop farmers, 27% of the populations were poor while among the livestock farmers, 38% of the populations were poor. The poverty depth of the crop farmers and livestock farmers was 0.072 and 0.098 respectively. This implies that they would need to be increased by 7.2% and 9.8% respectively for them to come out of poverty and become non-poor. The poverty severity measures the distance of each poor person to another. Among the crop farmers, the distance was 0.038 while in the livestock farmers the distance was 0.052. Overall, a comparison of the poverty status of the crop and livestock farmers indicated that the poverty status is relatively close even though it is higher among livestock farmers. The result may not be unconnected to excessive expenditure incurred by head of household as a result of increase household size and low-income occasion by subsistence nature of farming.
Factors Influencing Poverty Status of the Respondents
Table 3 shows the factors influencing poverty status of the farmers. The regression classification table revealed that the binary logistic model predicted 97% of the regression correctly. The model fits the data at (P<0.001) as indicated by the chi-square goodness of fit statistic (73.28). The goodness of fit of the model proved that the variables tested in this study were valid to explain the determinants of poverty in the study area. Besides, the Nagelkerte R2 value (0.867) shows that about 87% of the outcome (Likelihood of being poor) can be explained by the selected independent variables captured in the model (Table 3).
Table 2: Logistic Regression Result on Factors Influencing Poverty Status of the Respondents.

Percentage Prediction = 97.57%
Goodness of fit chi-square (df=11) = 73.28 (P<0.001) Nagelkerte R2 = 0.867
***, ** and * = figures significant at 1%, 5% and 10% levels respectively.
Source: Computation from field survey data, 2017.
The results of the regression model indicated that eight (8) of the eleven (11) explanatory variables influenced the poverty status of the farmers. The variables were age, educational level, and household size, farming experience, farm/herd size, household income, household expenditure and membership of cooperative. The coefficient of age of the farmer was significant and negatively related to the probability of a household becoming poor. This implies that the age of the farmers is a causative factor of poverty. As age of the farmers increase, the likelihood of being non poor is reduced. This conforms to a priori expectations and work by Ayalneh [15], Obiesesan [16], who opined that older households had greater likelihood of being non-poor. This may be attributed to increased experience and exposure to farming operations and management practices as their age increases.
A positive and significant relationship was found between educational qualification and the likelihood of being non-poor, hence, the higher the educational level, the lower the tendency of been poor. The result is in conformity to a priori expectations and work by Ogwunike [16] who found that a positive significant relationship existed between educational level and the probability of being non-poor. The coefficient of household size was negative and was significant at 1% level. This implies that, the higher the household size, the more likely to become poor. Ceteris paribus. This could be as a result of the fact that the members of such households would have to depend on the limited resources that is available to the household thereby reducing the per capita income of the household. This is in agreement to a priori expectations and work by Khan [5] and Ogwumike [16].
A positive and significant relationship was found between farming experience and the likelihood of being non poor at 5% level. This implies that the higher the years of farming, the higher the probability of being non-poor. This is in conformity to a priori expectations and work by Omonona [10] who stated that exposures and experiences gathered over the years help rural poor people to fight poverty. The author further opined that experience in farming help to reduce losses thereby encouraging proper handling and management of relatively scarce resources. There was a positive and significant relationship between farm/herd size and the likelihood of being non-poor. This implies that as the farm/herd size of the farmer increases, the probability of the household being nonpoor is increased. This finding conforms to a priori expectations and work by Eneyew [17] and Alemu [18] who found that a unit increase in land holding increased the probability of being nonpoor. The coefficient of household income was significant at 1% level and positively related. This implies that as the household income increase, the probability of being non-poor increases. This is in agreement to a priori expectations and work by Alemu [18] who found a positive relationship between household income and the likelihood of being non-poor.
In conformity to a priori expectations, the coefficient of household expenditure was negative and significant at 5% level. This indicated that, the higher the household expenditure, the lower the likelihood of being non-poor. Ogwumike [19] stated that, excessive expenditure by household head is a pointer to poverty. The coefficient of membership of cooperative was positive and significant at 5% level. This implies that, if a household head is a member of cooperative, the likelihood of being non-poor increases. This will not be unconnected with the fact that members of cooperative in the rural settings help their cooperative members in time of needs and also provide incentive and loan facilities to those in need.
Conclusion
The research has shown that, the incidence, depth and severity of poverty were high among the farming households even though some of the farmers fall above the poverty line. The study has also shown that the rate of poverty is relatively higher among livestock farmers compared to crop farmers. Meanwhile, the study has revealed that several factors influences the poverty status of the farming households such as age, educational level, household size, farming experience, farm/herd size, household income, household expenditure and membership of cooperative [20].
Given these findings, therefore, it is recommended that:
a) Government and other relevant non-governmental organizations should provide incentives and infrastructures that will enhance productive and sustainable agricultural development in the rural areas.
b) The farming households need to diversify their productive activities through mixed farming and value addition to improve their non-farm income thereby reducing poverty.
c) Policy makers and the operators of rural economy should carefully understand those variables that influence the poverty status of the farming households and address them critically and vigorously.
No comments:
Post a Comment